Today, everything is in data – and your success is there, too. However, it is not a trivial task to make it happen. But we have the expertise to help. No matter if you need insight into which potential your data holds, which important clues it hides or which opportunities can originate from it: We are happy to start or to assist with your journey in the world of data science. Be it a first analysis of available data, building mature data pipelines or even leveraging full-scale machine learning projects, the experts at Scandio can cover the complete life cycle of your projects.
-
Data Analytics
Any data-driven project relies on understanding the link of your applications and their respective data. We will start this endeavour with you.
-
Data Processing
For long-term storage and further analysis of your data, we build cloud-based data processing pipelines.
-
Modelling
With machine learning and models we optimize your processes and create data-based services to help you gain insights into your data.
-
Training
We create suitable cloud environments for model training including versioning of the training files and the emerging models.
-
Deployment
We not only set up the continuous delivery pipeline but also design the model to ensure scalability and continuous evaluation of the model.
No matter if you are taking your first steps in data science or already leverage fully fledged ML projects: we help you along the whole data science journey!
- 🚀
Start with analyzing the challenge
- 🚀
Follow up with a small focused team on a proof of concept
- 🚀
Scale the team to full size, target for a productive MVP, including operations
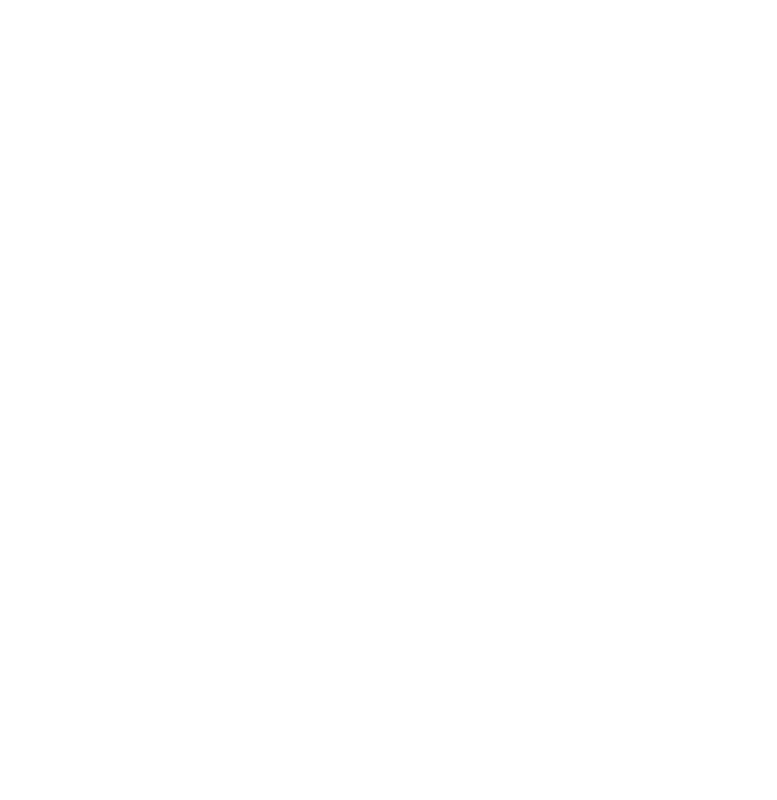
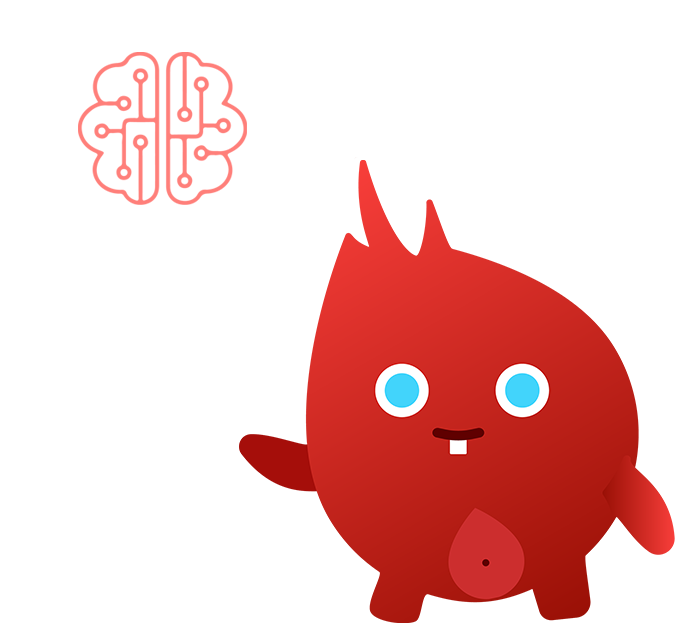
Success Stories
A selection of customer projects that highlight our competences better than anything else. Object Detection in FridgesChallenge
New fridges of a German home appliance manufacturer come equipped with cameras taking a photo every time the fridge door is closed. The users should be sent a notification when they are about to run out of common grocery items such as milk.
Solution
We developed state of the art object detection algorithms. We also aided in the collection and labelling of data, setting up data pipelines, as well as the deployment and tracking of the models in production.
Benefit
A machine learning use case is developed end to end and ready for the customer to bring into the market.
Used Technologies
TensorFlow, Python, Labelbox, MLflow, AWS
Challenge
Smart ovens receive forecasts on their remaining baking duration from AI models served in the cloud. A re-training on very large data amounts was to optimise user experience.
Solution
We enabled the re-training by supplying high quality training data extracted from very large data sets (>10 TB) via highly scalable data querying pipelines. Since deployment the new models are extensively monitored.
Benefit
The ML overall performance has been considerably improved and the user experience thus optimised.
Used Technologies
TensorFlow, Python, pandas, MLflow, AWS
Challenge
“Tracker” photo-voltaic systems are more susceptible to faulty mounting and component wear due to their moving solar modules. The data which is collected within these systems has to be collected to indicate these risks at an early stage.
Solution
A Grafana instance visualizes existing parameters in addition to forwarding anomalies to the responsible employees.
Benefit
The project presents a straightforward interface that provides an overview of the behaviour of all tracker plants. This gives the customer Schletter quick insights as well as immediate notifications when anomalies occur.
Used Technologies
Go, AWS, Grafana, Python, InfluxDB
Challenge
In 2019 a major household appliance manufacturer was looking for a way to deploy machine learning services for their productive use. The services needed to be highly scalable as well as integrated with extensive business logic.
Solution
We built a Kubernetes cluster utilizing Amazon EKS and Seldon Core, connecting machine learning models with business logic.
Benefit
We built an infrastructure that allows scalable and stable serving of models in production.
Used Technologies
AWS, Kubernetes, Python, Seldon Core, Terraform, Amazon EKS, Apache Flink, Grafana
Challenge
New fridges of a German home appliance manufacturer come equipped with cameras taking a photo every time the fridge door is closed. The users should be sent a notification when they are about to run out of common grocery items such as milk.
Solution
We developed state of the art object detection algorithms. We also aided in the collection and labelling of data, setting up data pipelines, as well as the deployment and tracking of the models in production.
Benefit
A machine learning use case is developed end to end and ready for the customer to bring into the market.
Used Technologies
TensorFlow, Python, Labelbox, MLflow, AWS
Challenge
Smart ovens receive forecasts on their remaining baking duration from AI models served in the cloud. A re-training on very large data amounts was to optimise user experience.
Solution
We enabled the re-training by supplying high quality training data extracted from very large data sets (>10 TB) via highly scalable data querying pipelines. Since deployment the new models are extensively monitored.
Benefit
The ML overall performance has been considerably improved and the user experience thus optimised.
Used Technologies
TensorFlow, Python, pandas, MLflow, AWS
Challenge
“Tracker” photo-voltaic systems are more susceptible to faulty mounting and component wear due to their moving solar modules. The data which is collected within these systems has to be collected to indicate these risks at an early stage.
Solution
A Grafana instance visualizes existing parameters in addition to forwarding anomalies to the responsible employees.
Benefit
The project presents a straightforward interface that provides an overview of the behaviour of all tracker plants. This gives the customer Schletter quick insights as well as immediate notifications when anomalies occur.
Used Technologies
Go, AWS, Grafana, Python, InfluxDB
Challenge
In 2019 a major household appliance manufacturer was looking for a way to deploy machine learning services for their productive use. The services needed to be highly scalable as well as integrated with extensive business logic.
Solution
We built a Kubernetes cluster utilizing Amazon EKS and Seldon Core, connecting machine learning models with business logic.
Benefit
We built an infrastructure that allows scalable and stable serving of models in production.
Used Technologies
AWS, Kubernetes, Python, Seldon Core, Terraform, Amazon EKS, Apache Flink, Grafana
Our Services in Detail
-
Big data in the cloud
- Developing data processing pipelines
- Data fusion (bringing multiple data sources together)
- Storing data in databases and data lakes (helping companies "where to put things")
- Evaluating data: (helping to make sense of what data companies have)
- Transform data (helping customers to put ideas into practical use)
-
Exploratory Data Analysis
- Analyzing the data
- Stating hypotheses for potential model development
-
Machine Learning Model development
- Setting up the necessary tools and services for model development
- Designing of ML model (coming up with an idea that might work)
- Training of ML models (carrying out this very idea)
- Assessing ML models (see whether it works as desired)
-
Serving models in the cloud
- Developing services to connect the data sources with the model server
- Creating the cloud infrastructure
- Setting up deployment pipelines
- Ensuring scalability
-
Monitoring models
- Designing ML monitoring solutions
- Advising with selecting third-party solutions fit for enterprise use
- Providing guidance on statistical matters
Our experts clarify the most common ideas about AI and Data Science in our glossary. Also understandable for non-techies. 😄